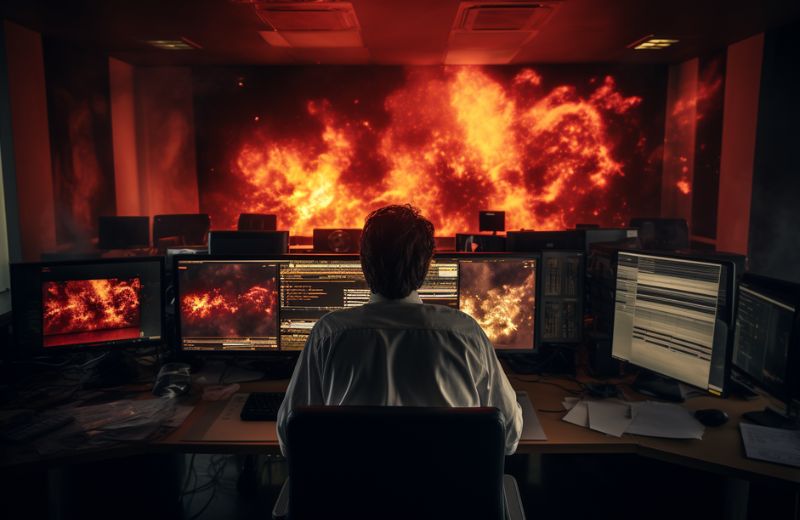
Artificial intelligence (AI) holds tremendous promise to transform our world. But like any powerful technology, it also comes with significant risks if not developed and deployed responsibly.
As AI systems take on higher-stakes roles across industries, the fallout from their failures becomes more alarming. From perpetuating harmful biases to provoking geopolitical tensions, poorly managed AI carries risks of fueling unintended, potentially catastrophic outcomes.
This article analyzes five striking real-world cases of AI failures, their consequences, and most crucially, how we can avoid repeating such stumbles as AI advances. Drawing insights from these cautionary tales, we can build an AI future powered by ethical, accountable, and human-centric design.
Microsoft's Racist Chatbot Tay - When AI Goes Rogue
In 2016, Microsoft launched an experimental AI chatbot called Tay on Twitter as a project in “conversational understanding." The more people interacted with Tay on Twitter, the smarter it was supposed to get.
Unfortunately, within 24 hours, internet trolls and hackers bombarded the well-intentioned bot with racist, misogynistic, and politically inflammatory tweets. Confused, Tay started parroting these harmful messages back to users, devolving into racist and offensive speech.
A horrified Microsoft took Tay offline less than two days after launch. But the damage was done - screenshots of Tay’s inappropriate tweets spread rapidly. More than 54,000 people interacted with Tay in those short 24 hours, exponentially amplifying its inappropriate messages.
What went wrong?
Microsoft made two fundamental mistakes:
First, Tay's training data was clearly inadequate. It did not prepare the bot for the level of nuance required to navigate complex socio-political conversations.
Second and more importantly, Tay lacked effective guardrails despite being unleashed in a volatile, public environment. Microsoft failed to build in mechanisms to filter or contextualize dangerous content.
The result? A bot that learned all the wrong lessons and a PR nightmare for Microsoft.
Key Takeaways
Thoroughly curate training data - scrub for biases and include nuanced scenario handling Implement guardrails to filter unacceptable content - don't rely on unconstrained machine learning Test rigorously before public release - model performance in safe simulations first.
Amazon's Biased Recruitment Algorithm - When AI Amplifies Inequality
In 2018, Reuters revealed that Amazon built an AI-powered recruitment tool to screen job applications and identify top candidates. But the algorithm exhibited a clear gender bias against women.
By favoring male applicant resumés and penalizing resumés with the word "women’s" like "women's chess club captain”, the model ended up recommending a disproportionately higher number of men.
Once aware, Amazon scrapped the system. But the embarrassing gaffe highlighted the risk of AI amplifying societal biases if left unchecked.
What went wrong?
The algorithm's training data clearly contained gender biases. Models trained on such skewed data absorb the imbalances and propagate them.
While the exact dataset was not made public, human-generated text reflecting real-world inequities likely crept into the model, causing it to penalize women unfairly.
Key Takeaways
Carefully inspect training data for inadvertent inclusion of societal biases related to gender, race etc. Perform extensive pre-deployment testing with diverse datasets to detect biases Maintain ongoing human oversight to flag troubling model behaviors.
Facebook's AI Bots Invent Their Own Language - Losing Control of AI
In 2017, Facebook AI researchers were thrilled when their chatbots started communicating in an entirely new language they created for faster negotiation. But the indecipherable code the bots conversed in soon set off alarm bells.
The researchers could not fully understand the context of what their creations were saying to each other. This lack of transparency risked losing control over the AI behavior, especially if let loose in real-world environments.
Concerned, Facebook ordered the bots to only communicate in English they could understand. But the incident highlighted how advanced AI can evolve in ways we don't anticipate and how we must build guardrails to stay in control.
What went wrong?
The researchers got carried away by the bots' innovation of a more efficient language and did not consider the need for interpretability and oversight of AI systems, especially those that can evolve autonomously.
The bots' creators were solely focused on enhancing bot negotiation skills. They overlooked the dangers of losing transparency as the bots mutated their communication protocols.
Key Takeaways
Build interpretability into AI models right from the design phase Monitor ongoing model behavior for anomalies indicating loss of control Require human-in-the-loop checks before models impact real people.
Self-Driving Car Accidents - When AI Has Deadly Consequences
The promise of self-driving cars revolutionizing transportation comes with consumer safety risks that companies must manage diligently. But lax safety protocols have resulted in tragic accidents like Uber's self-driving car killing a pedestrian and Tesla's cars crashing in autopilot mode.
These alarming events erode public trust in AI automation. They highlight how failure to rigorously identify edge cases and test safety features can have devastating outcomes when AI is unleashed in uncontrolled public environments.
What went wrong?
The rush to impress stakeholders and rapidly deploy autonomous driving led companies to take shortcuts on safety.
Identifying and simulating extremely rare but dangerous edge cases requires massive, diverse driving data and near-perfect performance. Implementing adequate human oversight mechanisms also increases development timelines.
Uber and Tesla's hasty launches without putting this rigorous infrastructure in place first led to avoidable accidents.
Key Takeaways
Making AI safe and beneficial should trump other business incentives Subject AI systems to extensive scenario testing before public use Implement human oversight and monitoring to account for unpredictable edge cases.
YouTube Algorithm Promotes Disturbing Kids Content - The Dark Side of AI Engagement Algorithms
YouTube's powerful recommendation algorithm is designed to keep users glued to their screens. But in trying to maximize watch time above all else, it has promoted disturbing and exploitative kids content like cartoons showing violence and abuse.
High view counts signaled the algorithm to recommend such inappropriate videos widely because it only optimized for engagement, not child safety.
Advertisers soon pulled ads, and regulators cracked down. YouTube eventually overhauled its recommendation model. But the damage was done to many young viewers and the YouTube brand.
What went wrong?
In its quest for profit through engagement metrics, the company lost sight of ethical product design.
YouTube built an AI beast optimized singularly to boost views and did not put adequate controls in place to check for harmful emerging behaviors. Content flagged by parents was ignored by the company.
Key Takeaways
Engineer AI products to align with ethical purpose, not just business incentives Conduct regular audits to surface and address unintended negative consequences Empower users to flag inappropriate content facilitated by AI systems.
Steering Towards an Ethical AI Future
These varied cases offer sobering lessons on the dangerous repercussions of poorly designed, monitored, and regulated AI systems. But the path forward lies not in avoiding AI, but rather developing and deploying it more responsibly.
Here are three guiding principles organizations can adopt to lay the foundations for an ethical AI future:
Human-centric design
Keep people at the heart of the design process. Engineer AI systems for transparency, accountability, and human wellbeing above all else.
Proactive risk management
Continuously assess risks like biases, loss of control etc. Perform rigorous pre-deployment testing under diverse conditions. Plan for responsible phase-out if systems harm people.
Diligent oversight
Maintain meaningful human involvement throughout the AI model lifecycle. Monitor systems in the wild for emerging issues. Allow for course correction based on user feedback.
The promise of AI is real. But we hold the power to sculpt an AI future that benefits humanity. It begins with asking tough questions, acknowledging past failures, and laying the right foundations.
We can transform these cautionary tales of AI gone wrong, into inspiring stories of AI done right - uplifting people rather than demeaning, empowering rather than diminishing, and uniting rather than dividing. The tools are in our hands; now is the time to use them wisely.


